Conceptual Architecture of Customer Data Platform (CDP)
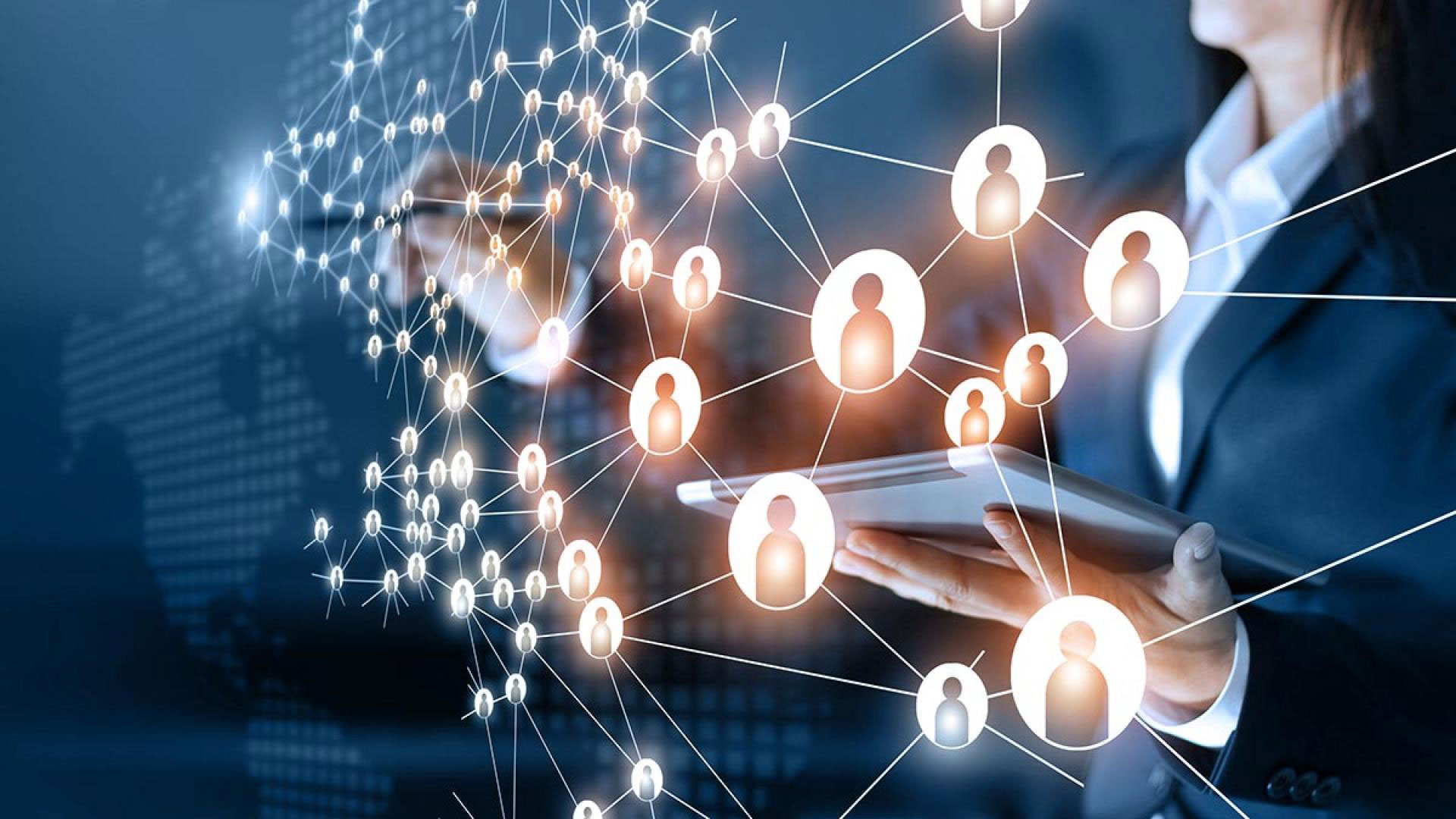
A customer data platform (CDP) is vital for businesses today. It brings together many dimensions of customer information so that a 360-degree understanding of a customer can be created and enriched with information from multiple sources. The final aim is to generate insights and AI models that can be used to drive better customer experiences, commerce (purchases, cross-sell) and operational efficiencies.
However, enterprises often face the following two challenges:
- CDP programs often tend to become too complex leading to delays in returning value to the business.
- The systematically deployment of AI insights into operations as part of closed loop analytics remains a challenge for the industry.
These challenges are a motivation for this post. The identification and implementation of a CDP does not have to be complex. In this post I wanted to present a bird’s eye view of some of the primary data and technical architectural components that business & technology leaders must think of as they embark on CDP programs – whether licensing a platform from a leading vendor or customizing an open-source product.
Conceptual Architecture of Customer Data Platform – Explained Below
A CDP can be thought of in terms of the following key functional blocks that span the overall data management lifecycle. It is helpful to think in terms of a functional architecture because it aligns the CDP more closely to its purpose.
Let’s go bottoms up and build it up to enable an easier understanding of the multiple layers of Customer Data Platform Architecture.
Level 0: The core data repository
This is the core Customer 360 vision for a CDP. Ideally the development of this level should be aligned with the benefits that we wish to drive through AI and better insights aka personalization, audience analysis, cross-sell, better conversions, lower stock outs, decrease locked inventory, etc.
This layer is often virtualized and consists of many functional blocks representing key data workstreams that will help us deliver the business objectives. For example, online clickstream data, transactions, purchase behavior etc. are often all part of this layer.
To enable this comprehensive repository, the integration architecture must support both real time as well as batch ingestion mechanisms. At this level we also have the challenges of customer identity resolution and master data management. As with any data management program, creation of this stage means external and internal source management, multiple levels of staging and cleaning layers, data quality, data masking, and so on.
Because of the inherent complexity of data management, this level can often overwhelm and dominate the scope of a CDP program. We must strategically define the (never ending) scope of Level 0 so that the business ROI can be generated in a timely manner. In other words, this layer must be incremental business value led.
Level 1: Insights Generation Engine
This architectural component is generally not considered as part of the core data repository but is critical to a CDP delivering the promised outcomes.
Typically, the analytical use cases here are defined with a view to the desired business outcomes. For example, one of the most common insights to be generated is about customer segmentation. Any subsequent models must keep the segmentation in mind. The failure to keep segmentation front and center leads to sub-optimal outcomes. For example, we could be running a campaign based on incorrect segmentation thus leading to product cannibalization in the market, or we could be trying to fix an operational supply issue without clear insights on whether the issues disproportionately affect any specific customer segment.
This level is also closely aligned with traditional business intelligence – the visualization of data according to the many slices and dimensions possible.
Level 2: Program and Campaign Management
This functional component of the CDP provides a structure to the campaigns and ensures that we do not create conflicts or overlaps between campaigns. This conceptual block is where most of the business facing programs are managed and executed. For example, we could be executing a cross-sell campaign and a product adoption campaign simultaneously, or premium brands could be running campaigns to the audience of a lower priced brand.
So, this component, although not purely a technical one, serves as a key governance tool. This level also flows directly into the management reporting on campaign performance and attribution.
We also often define the Level 0 or Customer 360 requirements at this level. That allows for the Level 0 layer to be built in an incremental and Agile fashion, with a view towards the business value expected.
Level 3: AI Operationalization
This functional block is the mechanics by which generated insights from Level 1 are integrated with either operational systems or customer engagement systems.
Failure to consider this component of the CDP holistically is one of the primary reasons that AI programs often fail to be systematically utilized in production operations systems.;
For example, as the insights on cross-sell targeting are generated and a campaign is kicked off, the extent to which this is automated and integrated with the eCommerce systems defines the success or failure of most CDP and AI programs. After all, this is the moment of truth. Insights implementation will depend on a variety of factors such as available budgets, technology constraints, or operational conflicts. However, partial versus full implementation must be analyzed and planned consciously.
Technologically insights generated from AI are consumed and utilized via various means such as an API gateway. This level also serves to build in the reverse data feedback loops to feed into level 0. When this cycle is optimized, then the closed loop analytics mission is properly met.
Level 4: Management Reporting
The final component in the conceptual architecture of customer data platform (CDP) is the generation of insights for decision making and control. This is where we generate metrics on sales performance, product adoption, etc. This level also has the traditional components of exploratory data analysis and hypothesis so that new ideas can be generated.
We cannot control and improve what we cannot measure. So, thinking of this component is critical right at the time of developing the capabilities of the CDP. Often the evolving requirements here lead to additional enhancements in the top to bottom CDP stack itself.
Conclusion
Today, a customer data platform (CDP) is a significant and vital investment for enterprises. However, when the Customer 360 mission overwhelms other components of the architecture, the vision of a CDP remains unfulfilled.
I hope that this blog helped you understand the key business components and conceptual architecture of customer data platform (CDP) program without getting bogged down by the technical details. Know more about AI led CDP for Retail.